Quantitative trading refers to strategies that rely on mathematical computations and data-driven algorithms (quantitative analysis) to come to trading decisions. Quantitative traders primarily use this form of trading in financial institutions and hedge funds.
As a result, the transactions are usually sizeable and often involve the buying and selling of a wide variety of asset classes (hundreds of thousands, in fact). These range from metals, stocks, currency pairs, etc.
Quantitative traders typically formulate a trading strategy and construct a mathematical model based on it. The team develops a software program to implement the model on historical market data.
The model undergoes backtesting and optimisation. If the results are promising, the team deploys the system in live markets using real capital.
The steps in quantitative trading
For quantitative trading to work, one must undertake several steps. These include the following:
- Step 1: Quantitative traders begin by collect data from the financial markets to analyse patterns and/or correlations. Examples include earnings reports, price data, economic indicators, and more. It may also include sentiment data (data derived from news publishers, social media, etc).
- Step 2: Analysts examine the data using various statistical techniques, such as correlation and regression analysis, time series analysis (methods like ARIMA and GARCH), hypothesis testing, and probability distributions, among others. This is to identify analyse and sport patterns or trends, as well as factors that may impact future price movements.
- Step 3: After completing the statistical analysis, analysts build mathematical models to predict the behavior of different financial instruments. To ensure reliability and suitability for live trading, they test and backtest the models.
- Step 4: The team converts the models into algorithms for automated trade execution. The system executes the trades based on predefined rules or criteria, typically at speeds that no human trader is likely to achieve.
- Step 5: Quantitative traders depend on sophisticated trading systems to carry out their trades using the algorithms. These systems frequently connect directly to exchanges or trading platforms, ensuring that they execute trades instantly. This is particularly important for trading strategies like arbitrage, where price discrepancies between markets might exist, albeit for just milliseconds.
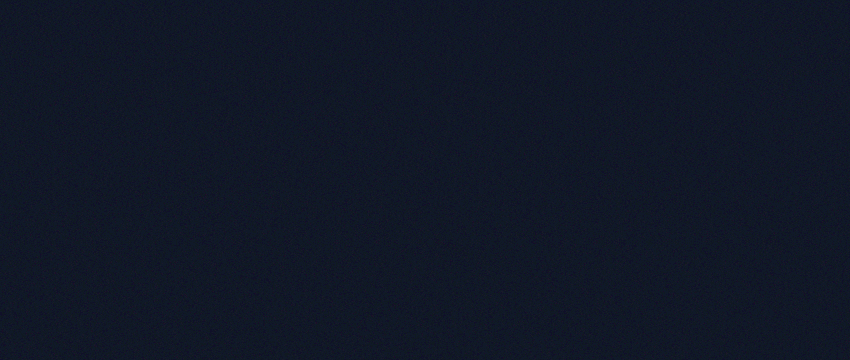
What is required to become a quantitative (quant) trader?
Becoming a quant trader is something that requires specific skills and expertise. A keen interest in mathematics is helpful. Experience with statistical analysis, data mining, and research methodologies is also valuable.
A quant trader is also someone who is familiar with automated trading systems. A quant trader often holds a degree in financial engineering, computer science, applied statistics, or mathematical modeling.
The appeal and challenges of quantitative trading
There are several reasons that quantitative trading is appealing to certain groups of traders. For one, while a typical trader can monitor, analyse, and make decisions on a set number of trades, the challenge of having to wade through overwhelming amounts of market data often hampers sound decision making, resulting in poor trading outcomes.
Quantitative trading can help reduce this limitation by using computers to automate this process, including the execution of trades. This in turn removes the stress that comes with trying to leverage a large volume of trading opportunities to maximise potential gains.
Which leads us to the second reason that quantitative trading appeals to traders, and that’s the elimination of emotional bias. By relying on computers and mathematical algorithms, traders make decisions based on logic and data rather than emotions like anxiety, fear, and greed.
Traders can also apply quantitative strategies across different financial instruments and markets simultaneously, allowing for effective portfolio diversification.This in turn helps reduce the risk associated with focusing on a single asset or market.
By trading multiple instruments using quantitative strategies, traders spread risk more evenly, minimizing the impact of adverse market movements and optimizing overall portfolio resilience. Quantitative models also allow for scaling to trade larger volumes, creating a more efficient approach for managing large portfolios.
The challenges of quantitative traders
This is not to say that quantitative trading doesn’t come with its own set of challenges. It absolutely does.
- Financial markets are constantly evolving, making it essential for quantitative trading models to adapt to changing conditions. Many quantitative traders design models that perform well in specific market environments but may struggle or fail when those conditions change.
- The quality of data is also very important as it is what quantitative strategies depend on. Poor quality data (i.e. inaccurate or incomplete) can result in faulty models, leading to poor trading decisions and negative trading outcomes.
- As with all trading strategies, quantitative trading is also at risk of being impacted by unanticipated market moving events, be this market crashes, shifts in liquidity, etc.
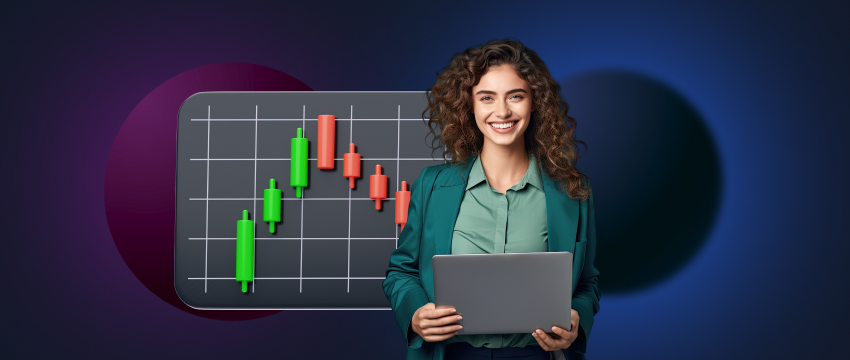
Managing risk from quantitative traders
Essential techniques for managing risk in quantitative trading include:
- Before implementing a trading strategy, quantitative traders may be inclined to conduct backtesting to assess its historical performance. Stress testing involves subjecting the strategy to severe market scenarios to assess its resilience. These tests enable traders to identify vulnerabilities and refine their strategies before committing actual funds.
- Setting stop-loss and take-profit orders to reduce losses that may be incurred and to lock in potential gains. These are typically triggered when predefined levels are reached.
- Focusing on position sizing, i.e. the amount of capital allocated to a position. Algorithms can be used to calculate optimal position size, reducing the risk of exposure and increasing the potential for making a profit.
- As we’ve already mentioned, portfolio diversification is another way to manage risk. It’s the cliché not putting all your eggs in one basket, and instead spreading your money across different assets so that if one trade performs badly, the remaining trades will act as buffer, safeguarding your funds better.
Becoming a quantitative trader
If you’re considering becoming a quant trader, you must make sure to learn the basics about financial markets, trading strategies and risk management.
This is of utmost importance before moving onto the more complex components of quant trading. Familiarise yourself with key concepts and terms associated to this type of trading, and consider taking a programming course, for e.g. Python, for data analysis and algorithm development.
Consider signing up for a demo trading account to manually test simple quantitative strategies on historical data to get a better idea of how they work.
Think about joining an online community or forum and share knowledge and experience with other quantitative traders. Gain as many insights as you can so that you can make more informed trading decisions when executing trades.
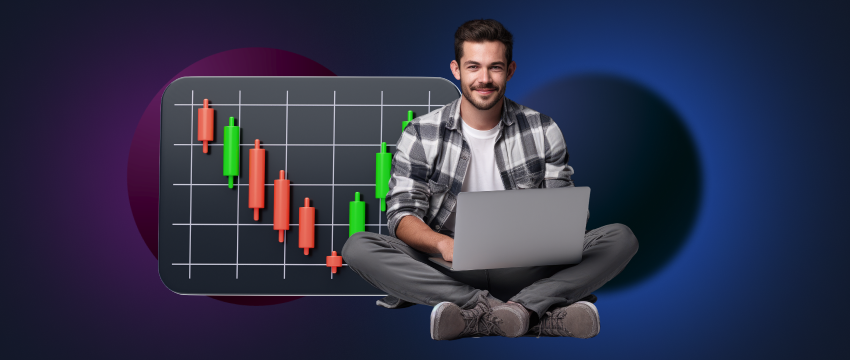
How quantitative traders work with T4trade
T4Trade is a popular global broker with clients worldwide. The CFD broker offers top-tier, 24/5 multilingual customer support, cutting-edge trading platforms, and flexible trading conditions.
T4Trade is also a great go-to resource for traders looking to learn more about forex trading in a user-friendly way.
A variety of videos, podcasts, eBooks, webinars, and videos-on-demand are curated by in-house specialists, catering to all types of traders.
T4Trade traders can also choose from a wide range of trading instruments across 6 asset classes, and enjoy flexible leverage, competitive spreads, fast trade execution and seamless deposit and withdrawal options.
Traders can also choose from multiple trading accounts that best suit their needs and individual preferences.
Disklaimer: This material is for general informational and educational purposes only and should not be considered investment advice or an investment recommendation. T4Trade is not responsible for any data provided by third parties referenced or hyperlinked in this communication.